Machine learning for building stock modelling
A method to map buildings through time with machine learning and aerial photos
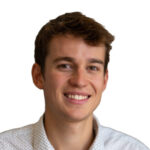
Francis Barre
Scientist / PhD Candidate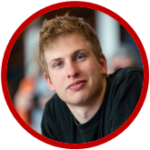
Nils Dittrich
PhD Candidate – NTNU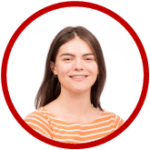
Zoé Cord’homme
Research Assistant – NTNUIndependent machine generated data
Local authorities use orthophoto projects for purposes like urban planning and forestry.
Timeseries with rich history
These orthophotos are available since the advent of cheap photographic film – reaching back to 1935 in some places in Norway.
Coverage follows human settlements
Most (historic) projects center around the built environment of municipalities.
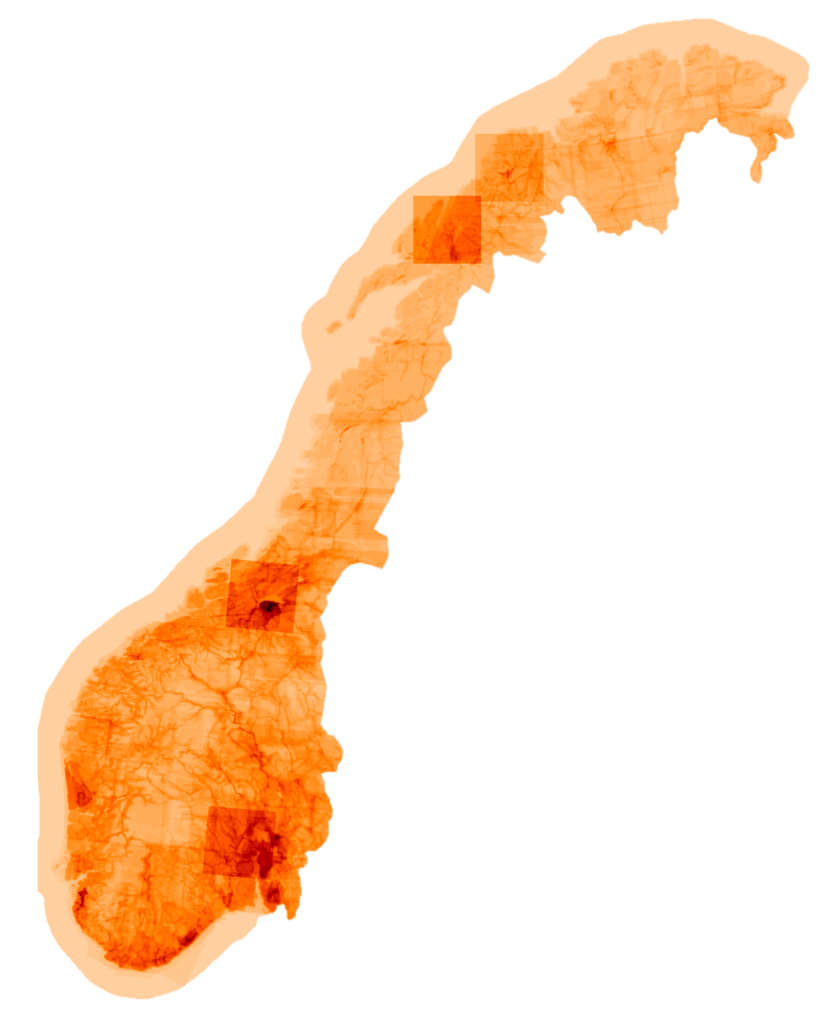
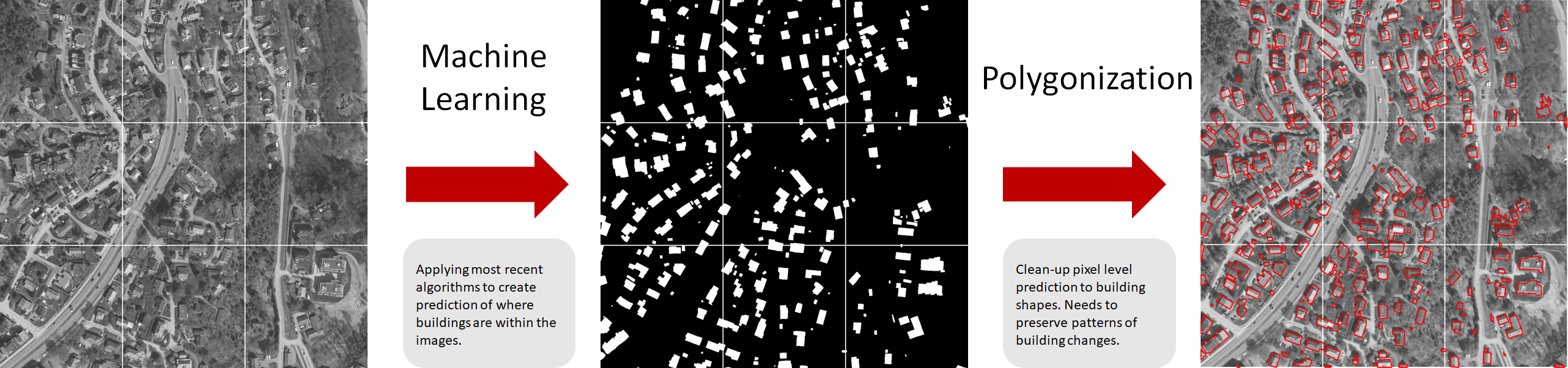
A building segmentation model was trained on the Norwegian dataset, using the most recent aerial photos combined with the cadastre data as a label. The model was then used to detect buildings on older photos, to provide the building stock in Norway for the last decades.
The building locations for different years allows for several studies:
- Analysis of demolition and renovation patterns
- Impact of natural hazards on the build environment
- Mapping urban development and land use from buildings
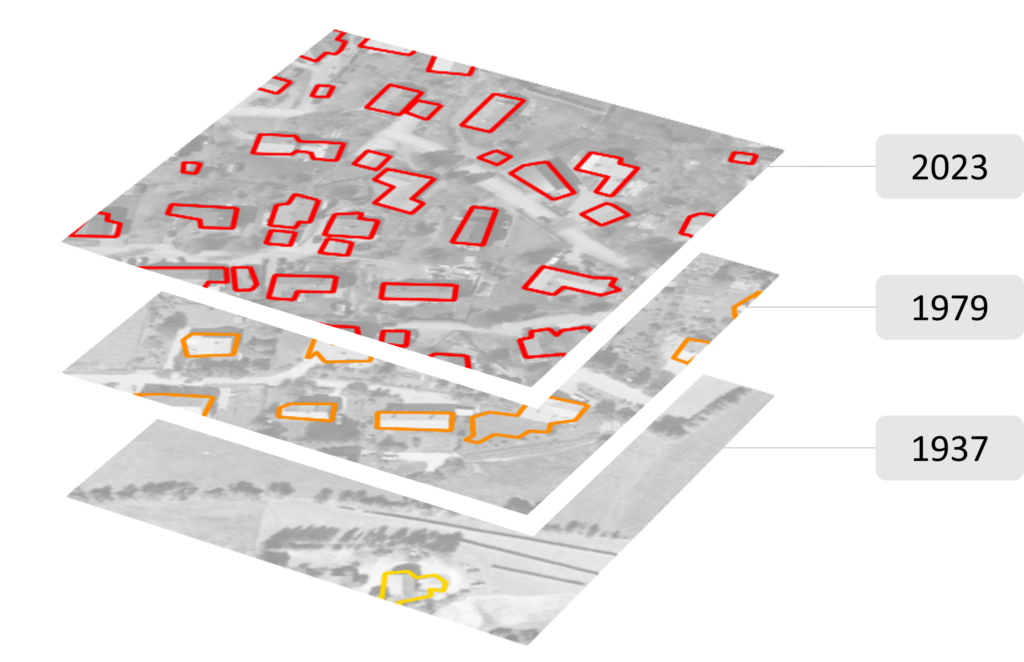